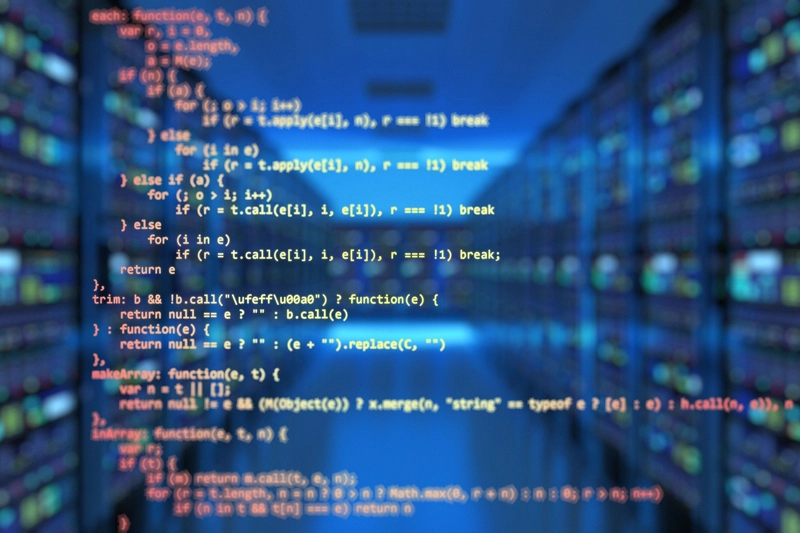
Data Talent Spotlight: Data Scientists
Data pervades every aspect of modern life. We generate massive amounts of data every day, and this data holds valuable insights that can help businesses make better decisions, improve their products and services, and understand their customers. But this data …
Data Talent Spotlight: Data Analysts
What is a Data Analyst? In today's digital age, data is everywhere. From social media platforms to e-commerce websites, businesses have access to vast amounts of data that can provide valuable insights into their customers' …
Building feature engineering pipelines
So you've exhausted all of your sources of data, but your model performance is still lagging. You could of course start tweaking parameters, but why not build a solid feature engineering pipeline?
Many of the businesses we work with have faced the dark side of Excel. A side which comes in the form of bloated and unsustainable Excel monster-books.
A summarized list of data science concepts
As a fun exercise, our team of Datakick Collaborators spent time discussing and collating a list of data science concepts.
More from our Blog
Want even more content? Take a look through our past blog posts.
Data science initiatives for your organization
Many industry leaders have moved beyond initial adoption and are now demonstrating and promoting real value from their data science efforts. But at the same time, many are still struggling to take the first step. So towards that, we have outlined a set of early-stage data science initiatives. With each being built around the people, data, and analytical processes of an organization.
Skills for deploying data science solutions
The role of a data scientist is evolving. And it's now more important than ever, that data scientists know how to deploy and scale their solutions for use across the wider organization. So, as a data scientist, how should you prepare yourself to confront these challenges? Which skills should you embrace and develop, versus which should you suggest are better suited for a dedicated data engineer or software developer?
The data science workflow
The data science workflow involves more than just fitting models. The problem needs to be appropriately framed, a good amount of effort needs to be spent on obtaining and preparing the data, and even after the modelling solution has been developed, there is still a piece of work around deploying and making the solution ready for use.